As one of the world’s oldest strategy board games, chess traces its roots to early sixth century India where it was known as chaturanga. Over the centuries the rules of the game evolved constantly until they were standardized somewhere during the 19th century. Chess had always been thought of as an intellectual man’s game; an amalgamation of quick thinking, keen awareness and deep understanding of what strategies and moves to employ, as well as when to employ them. Mastering these elements is key to becoming a chess grandmaster, and it would take years, even decades, to achieve this feat.
However, that would all soon change as AlphaZero, the Artificial Intelligence (AI) creation of Google’s DeepMind programmers, had learned to master chess in a mere four hours. Later that year, AlphaZero annihilated Stockfish, the leading go-to training tool for a majority of the world’s best chess players, winning 28 and drawing 72 of its 100-game closed door match. As for losses, much like Floyd Mayweather’s record, it had none.
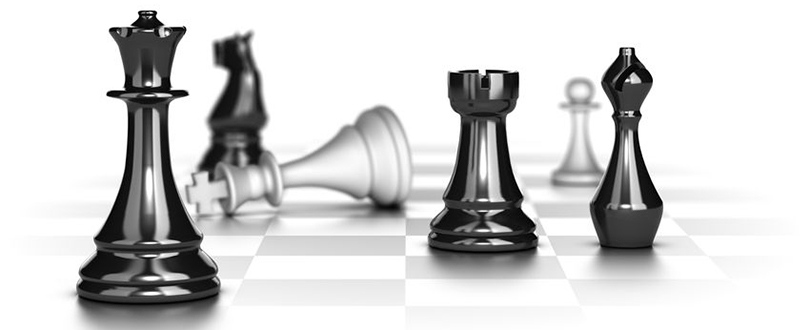
In the following year, DeepMind and Facebook began working together to master Blizzard Entertainment’s immensely popular real-time strategy game Starcraft II. But rather than personally programming and teaching the AI, both companies opened the datasets to the world, allowing the public to use the data to train the AI’s deep learning software.
Whether it’s called crowdsourcing or global effort, this was the best way for Starcraft II’s AI to grow due to the game’s complexity. Whereas in chess you only had six different pieces, each of which with their own unique moves, each of Starcraft II’s three factions had between 15 to 18 different “units”, some of which had up to four unique “abilities”.
Chess is turn-based and requires an AI to move the various pieces in a small, tightly defined grid of squares. In Starcraft II, a player could control hundreds of units with varying functions like builders and warships across a vast virtual geography. This includes resource management, forward planning, anticipating opponent strategies and be ever ready to change those dynamics in the blink of an eye. As of now, the AI is still struggling to master Starcraft II but both DeepMind and Facebook have been releasing a constant stream of updates to further improve the AI’s performance.
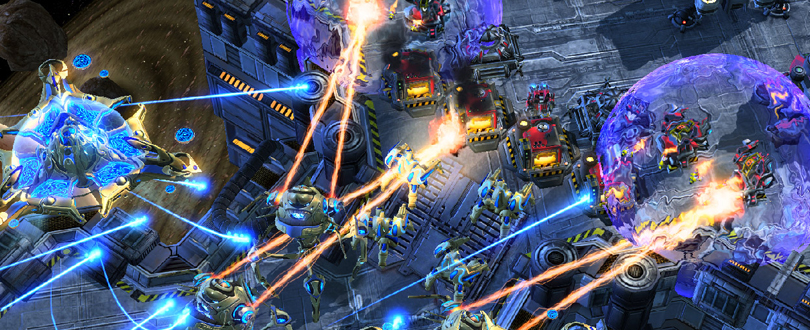
At the same time, the Elon Musk-backed project OpenAI surprised the world when it wiped the floor with professional esport players at Dota 2, an extremely popular multiplayer online battle arena (MOBA) game, in 1-on-1 matches. And it did this in less than 10 minutes.
In preparation for The International, the world’s biggest esports event, OpenAI trained by simply playing against itself for weeks. It wasn’t thought any strategies nor did it learn anything from human experts. From the moment of its conception, OpenAI started from complete randomness, making small improvements along the way until it eventually reached the level of a professional DOTA 2 player. Throughout the event, no one could beat it.
While this is a huge milestone, Dota 2 is not played as a 1v1 single-player game but a 5v5 team of five players strategizing to complete countless tasks simultaneously to achieve victory. A 1v1 battle in Dota 2 is nowhere as complex as the traditional 5v5 battle. However, it didn’t take long for OpenAI’s software to mature this year at the same esports event where it emerged victorious in a full five-on-five game against human opponents. Again, it learned everything on its own through experimentation at a rate 180 years per day.
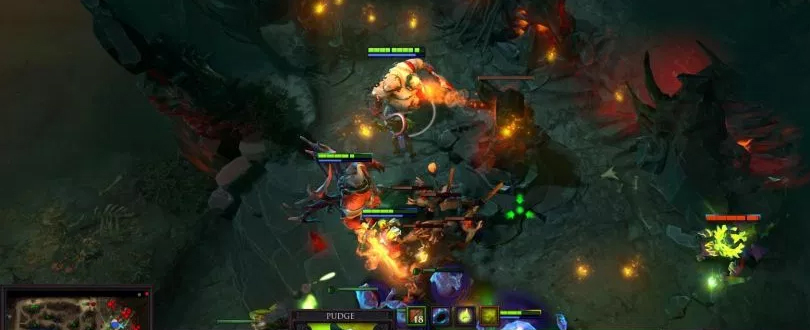
Of course there were a few conditions to make it more manageable for the AI such as a smaller pool of characters it can use and item delivery couriers that cannot be killed. Regardless, these minor adjustments did little to undermine its spectacular achievement. The professional human players it went against could not even progress far enough to pose a threat as the AI obliterated them early on in the game.
Yet, the results went differently once the constraints were removed. With the full character roster available and a vulnerable courier, the AI showed its limitations as the machine learning was lacking a long term game plan. The general consensus was that the AI played admirably well but not exceptionally so. As the match dragged on, the AI had trouble structuring a system that worked over a longer period. Despite its losses, the way OpenAI’s bots handled the game was still considered a victory for AI.
At the same time, Google’s DeepMind refuses to back down and was learning teamwork by playing first person shooter Quake III Arena’s Capture the Flag mode. The AI named For the Win (FTW) went through 450,000 matches of training multiple independent agents to take collective action that would benefit the team. From there it established an understanding of how to efficiently work with other machines or humans.
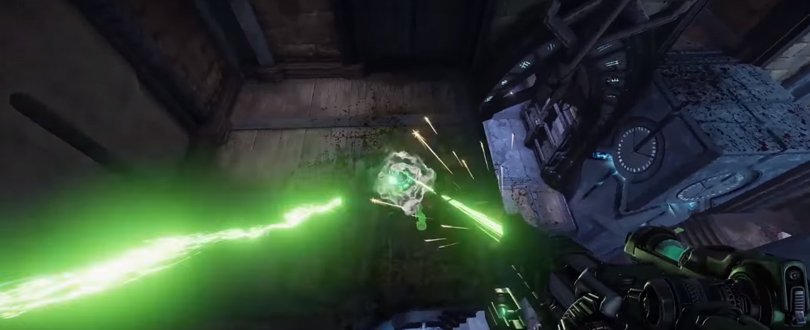
Just to give an idea on how much DeepMind’s FTW had grown; in a Capture the Flag tournament with and against 40 human players, the AI went undefeated when pitted against human-only players. Even if the human players adopted one machine partner in the team, FTW still stood a 95% chance of winning.
While watching AIs going against human is exhilarating, these exercises and experiments were more than just trying to beat professional esport players. It was about giving AI the ability to make a lot of micro decisions that would lead to a larger end goal. Digging deeper into AI gaming, it is obvious that the things learnt through video games have little use in the real world. However, the underlying methods invented for playing or winning a game can be invaluable when applied to real life.
When a collection of individual components come together, it paves the way for AI researchers and developers to build an adaptive and intelligent system that can benefit the world. This is when an AI evolves into an integrative AI, allowing people to apply its functions and services to robotics, Internet of Things devices and autonomous vehicles. From first learning that a queen can move all the way to the end of the chess board, this type of AI’s capability to learn could very well be the next big thing that propels us further than we can expect.